Is Tesla a car company or a new data-driven driving experience company? Is GE Aviation a big data analytics firm or a aircraft engine provider? Is Google a search engine or a integrated “everyday productivity enhancing” company? Is Facebook a social network or an engagement platform? Is Kayak/Priceline a travel aggregator or search analytics firm?
We are in the decade of data. I believe that data is fueling the Insight Economy. Every major industry is facing a similar trajectory: data volumes from many sources in multi-structured formats are exploding, and the market leaders see value in asking bigger “data driven business process-centric” questions of that data in real time.
Pattern detection, personalization, visualization, test-and-learn experimentation via rapid prototyping is the new norm. As part of that goal of finding better answers, deeper and clearer insights helps isolate trends which are invisible in a surface analysis, and the powers to experiment, to iterate quickly, and to explore are key in discovering those otherwise unnoticed elements.
Insightful “sense-and-respond” strategies coupled with new big data technologies are shifting “cost-to-serve” competitive boundaries and also shaking up “what is possible”. In parallel, developments in mobile, social and cloud technology are converging to create new opportunities for growth and business model transformation. This is causing industry boundaries to rupture as new innovation changes the way companies operate – reliable, insightful, actionable and faster analytics that help drive good decisions. For example, Facebook Programmatic Mobile Ads in 2 years does more ad business than Time Warner across all its properties. The pace of change is staggering.
I am convinced that analytical insights coupled with business processes, and Mobile Technology – (action enabler and insight consumption channel) will profoundly change consumer behavior and the basis of competition. All customer touch points (loyalty, e-mail, web, social, e-commerce, coupons) are converging on the mobile phone.
A whole new form of self-service consumer engagement enabled by analytics (discover, analyze, visualize, explore) is just starting to take shape.
The Race to World-Class Business Analytics
“Anyone who wants to keep their finger on the pulse of how a company is operating is investing in advanced analytics,” Celestica CIO Mary Gendron.
According to MIT research, top performing firms (world-class) tend to leverage analytics 5 times more than others.
Interesting these firms are leveraging analytics to change-the-business or drive improvements in customer experience. Most of the traditional firms tend to use analytics primarily to run-the-business (mostly reporting) vs. change-the-business?
World-class companies excel because they’ve made tough decisions about which analytical processes they must execute well, and they’ve implemented platforms need to streamline those processes. Result? Their platforms have become an asset rather than a cost, and tend to foster new experiences for customers.
In a world where customers have become increasingly social and mobile, world-class companies are taking bolder steps. They are rethinking story-telling, customer intimacy, customer experience, social co-creation, to define their path to a truly “real-time enterprise”——one that redefines interactions and relationships not just in the B2C space, but also in the B2B domain.
Leading World-class Analytics
While everyone is racing to create better analytics, there are still questions around data leadership, role clarity and priorities along the data supply chain…. (Raw Data -> Aggregated Data -> Contextual Intelligence -> Analytical Insights (reporting vs. prediction) -> Decisions (Human or Automated Downstream Actions).
According to IBM, 25% of today’s businesses now employ a Chief Data Officer – yet many questions still remain around how this role should be structured. Research has shown that the priorities for Chief Data Officers include:
- Finding Ways to Leverage and Monetize Existing Data
- Augmenting Data by Combining Internal and External Sources
- Data Quality
- Governance
- Data Privacy and Security
Examples of World-Class Analytics
Consider real-world analytics example at Netflix, UPS, Ford, British Airways, Priceline/Kayak.
Every single example is about competing and winning in a data driven economy where customers are in control and switching costs are very low, customer intimacy, customer experience are more critical than its ever been. Business must continue to push the man+machine data envelope and continuously look for patterns around key business questions. If they don’t they will be overtaken by competitors and will cease to matter or even exist (e.g., Netflix vs. Blockbuster or Apple vs. Nokia).
Netflix and Consumer Big Data Visualization
Netflix is reinventing television. With 50 million subscribers, Netflix is a major player among media and broadband companies. “We provide streaming services so people can watch whatever they want whenever they want” said Blake Irvine, a manager in the Data Science & Engineering group at Netflix.
With 300 billion data pipeline and streaming events a day and 10 billion rows of data per day, NetFlix has a lot of customer interaction data. Netflix relies on data to make decisions ranging from buying and recommending content, and improving the streaming experience on devices.
Happy customers consume and pay more. Netflix uses Tableau Software for visualization and storytelling….basically help them make sense of their plentiful and ubiquitous data. According to Albert Wong, a manager in the Netflix Cloud and Platform Engineering group, “We had gigabytes of data coming in every second. We were filling up disk drives faster than we could set them up. Cloud storage helped them keep up with their storage needs and data visualization tools like Tableau allowed them freedom of choice in connecting to different data sources. See this posting for more insights on Netflix architecture evolution.
UPS, Telematics and Analytics
UPS sees analytics as a key differentiator in their operational improvement journey. With global 24 x 7 x 365 e-commerce growing exponentially, UPS has over 55,000+ delivery trucks in the US alone and around 106,000 drivers, globally. UPS delivers more than 20 million packages daily. When you consider the fact that every driver at UPS has millions of ways to run their delivery routes, the number of possibilities increases geometrically. However, not all of these routes are necessarily optimal in terms of time, fuel efficiency and distance.
A reduction of one mile per driver per day translates to savings of up to $50 million a year. To focus on ways to reduce driving miles, UPS created the ORION (On-Road Integrated Optimization and Navigation) initiative.
The question becomes: how does UPS mine the sea of data from sensors, GPS, traffic and vehicles to arrive at the most effective route for drivers? Shaving a mile here and there can add up to big savings in fuel costs. The results are pretty amazing:
- UPS reduced 85 million miles driven per year. That equates to >8M fewer gallons of fuel used.
- Able to reduce engine idling time by 10M minutes. This led to savings in fuel consumption – around 650,000 gallons – and reduced carbon emissions by over 6,500 tons.
Calculating the costs of last-minute request changes from customers is the next frontier. Optimizing the supply chain using real-time actionable insights about customers, drivers, trucks, airplanes is the next frontier. Directed Actions from machine intelligence is the next frontier.
Ford and Analytics
Bill Ford: “The car is really becoming a rolling group of sensors.” So Ford like Mercedes, BMW, GM, Toyota, Lexus and others investing heavily in technology required to continually collect, extract, store, refresh, and present data.
The challenge facing automakers is to manage and efficiently analyse vast quantities of data, from sources such as vehicle error memories, dealer feedback and repair reports, to obtain meaningful data to feed the service and improvement process. The goal is to prevent failures by using predictive root-cause analysis on machine data.
At Ford:
- Digital Analytics and Optimization team has full responsibility for all B2C channels and N. American business units
- Dynamic multichannel testing and targeting with automation and integration of SEO/SEM, CRM, email, media, etc.
- Hyper-local dealer support digital algorithm delivered 85% increase in action rate and 48% decrease in cost per action
The car increasingly employs machine learning to glean and drive insight. It ingests data from both inside and outside the car, executes predictive models, and provides real-time access for Directed Actions. With next generation cars having 4G built in, we are about to unleash a tidal wave of data to and from the car.
British Airways “Know Me” Project
British Airways (BA) has focused on competitiveness via customer insight – what will the customer want or expect next? It has petabytes of customer information from its Executive Club loyalty program and its website. BA decided to put customer big data to work in its Know Me program. The goal of the program is to understand customers better than any other airline, and leverage customer insight accumulated across billions of touch points to work.
BA’s Know Me program is using data and applying it to customer decision points in following ways:
- Personal recognition—This involves recognizing customers for being loyal to BA, and expressing appreciation with targeted benefits and recognition activities
- Personalization — based on irregular disruptions like being stuck on a freeway due to an accident – A pre-emptive text message… We are sorry that you are missing your flight departure to Chicago. Would you like a seat on the next one at 5:15PM. Please reply Yes or No.
- Service excellence and recovery—BA will track the service it provides to its customers and aim to keep it at a high level. Given air travel constant problems and disruptions, BA wants to understand what problems its customers experience, and do its best to recover a positive overall result
- Offers that inspire and motivate—BA’s best customers are business travelers who don’t have time for irrelevant offers, so Know Me program analyzes customer data to construct relevant and targeted “next best offers” for their consideration.
The information to support these objectives is integrated across a variety of systems, and applied in real-time customer interactions at check-in locations and lounges. Even on BA planes, service personnel have iPads that display customer situations and authorized offers. Some aspects of the Know Me program have already been rolled out, while others are still under development. Early results are positive; customers have expressed pleasure at BA’s understanding of their needs.
Online Travel – Kayak/Priceline, Expedia, Google Travel
As travel becomes commoditized, customers need more analytical help in navigating through the options.
Priceline, Orbitz, Expedia, Google and others rely heavily on analytics for both internal decisions and customer offerings, and employ a variety of big data technologies. Expedia handles over a billion searches each year, so it has plenty of data to analyze. In terms of customer offerings in search, there are more analytics required in search and result rankings for hotels. Unlike airline flight results, which are displayed in order of price, hotel rankings consider variables such as customer’s preferred distance from the city center, ratings from TripAdvisor, facilities, and room pricing deals compared to alternatives.
In air travel search, Kayak/Priceline like others uses analytical models to ensure that prices displayed on its website are consistent with those on airline sites, since there are sometimes synchronization issues across data sources. Priceline also has a feature called flight price forecasting, which predicts whether the price of a particular flight will go up or down in next seven days. It also provides a statistical confidence level behind the prediction.
Kayak/Priceline makes extensive use of randomized A/B testing on its website to optimize “look to book ratios. Every day between 30% and 50% of users are participating in some type of test. Such testing is the only way to establish cause-and-effect relationships behind which features of the site lead to better results.
The look-to-book ratio is a figure used in the travel industry that shows the percentage of people who visit a site compared to those who actually make a purchase. This ratio is important to Web sites for determining whether visits are translating into purchases. To improve their look-to-book ratios, web properties resort to offering incentives such as naming your own price, “special” deals, providing live agent chatting, and enabling co-creation … TripAdvisor style forums to showcase travel experiences.
*****Analytical Fact ****
The traditional boundaries are merging. Take for instance retail, especially teen and fast-fashion retailers, where the velocity of digital customer engagement—through likes, comments, Instagram or Pinterest shares—has increased multi-fold in 2014.
The strategic focus today is not just generating insight but targeted and directed actions centered around, “How do you uncover patterns and anomalies? How do you help the retailer stand out in a crowded bazaar? How does a retailer stand out in the endless aisle of digital retailing? How does a retailer differentiate and compete for the shopper’s attention in the noisy saturated retail market?”
*****Analytical Fact ****
Analytics Driven Business Transformation
Multi-tasking customers have declining attention spans. If they don’t see something interesting or attention grabbing they quickly move to something else. Smartphones makes this switching behavior more acute.
Customer expectations about novel user experiences are rising quickly in all online segments. Simply meeting these enhanced expectations can be a major effort for organizations that are not analytical. For instance, retailers may need to step up their development of analytical platforms aimed at recommendations or next best offers. Banks, insurers, and telecommunications players may need to automate end-to-end sales, marketing and service processes so that customers can interact with the company in real time in an error-free digital environment.
IBM in their 2014 investor day summarized the new data value chain.
Digitizing Experience, Operations and Supply Chain
As human behavior gets more mathematically expressed, analytics is becoming integral to next generation customer facing Digital Products and Decision Processes.
Adoption of digital channels is changing the customer experience and lower costs. Digital especially multiscreen is forcing a new set of audience and user experience requirements – push vs. pull, mobile first, social graph, same UX across all screens. Also driving a fundamental need for better apps – Easy to understand, easy to use; industry and task specialization and feedback driven.
All this is data driven. But data is necessary but not sufficient, it’s understanding and leveraging the signals in the data. Signals are the patterns extracted from data flows. They consist of patterns that are valuable in predicting or describing events. Data for pattern analysis come from transaction systems, CRM platforms, social media feeds, websites, blogs, and other data sources that exist inside and outside of organizations.
So analytics is a new growth enabler that integrates the company’s digital assets, software and services across digital marketing, mobility and transaction platforms to unleash the power of “man+machine” insights to drive growth and create new sources of value.
Why the emergence of a new platform? We are moving to a world of the datafication of everything — increasing the leverage of a range of information to provide new services, operate more efficiently, and market more effectively. Datafication without a proper “noise to signal” converter platform is chaos and a lot of useless data points.
But what is the big new new thing that causing a massive wave of investment in Analytics, Big Data capabilities and competencies? Better enhanced Customer Engagement/Experiences in the mobile world. To achieve long-term success, every business must go beyond “web” and transform customer experience while lowering cost-to-serve.
A example of signal detection (fraud detection and customer fading use case) is shown below (courtesy of Opera Solutions).
Revenue Growth question: How to meet and exceed customers’ expectations?
“We need to get customer insight to the front lines to reduce customer churn, not just in some exec’s inbox in a PDF report, or worse on a never-seen real time dashboard.” — Anonymous
Customers are being educated by e-commerce leaders like Amazon, user experience leaders like Apple and Google to expect an “ultraconvenient” experience, personalized in real time. New players in many industries are differentiating themselves from incumbents through convenience and service. Digital finance company Wonga, for example, settles loans in 15 minutes.
The bar is high for attracting and delighting customers in a digital world. Often, doing so requires investment in sophisticated big-data capabilities that use social, location, and other data, for example, to attract potential customers to product promotions at stores in their vicinity. Connect all your apps. Connect all your devices. Connect all your customer data.
Revolutionize the customer experience. I love this line from Salesforce.com…. “Welcome to the Internet of Customers. Behind every app, every device, and every connection, is a customer. Billions of them. And each and every one is speeding toward the future.” I am convinced we are going to spend the next 20 years perfecting this vision of an integrated, end-to-end digital capability to compete in a fast-changing business environment.
A parallel question: How is technology enabling or shifting customers’ expectations in a digital world?
According to Peter Norvig, Chief Scientist, Google “We don’t have better algorithms than anyone else. We just have more data.”
Technology waves like mobile, social, predictive analytics, wearable computing have changed customer expectations. Changing customer expectations have in turn impacted the data collection and aggregation process to support decision making.
Decision making is being enabled by various apps that produce and dashboard data… Systems of Record (ERP, CRM, SCM etc.) and Systems of Engagement (Marketing, Sales, Service)….Systems of Things (RFID tags, Mobile devices, Sensors etc.)
- Wave 1 was Decision Support Systems and Expert Systems.
- Wave 2 was Reporting and Business Intelligence (BI)
- Wave 3 is Descriptive and Prescriptive Analytics
- Wave 4 is Big Data, Cloud, Predictive Analytics, and Cognitive Computing
BI is about “sense and respond.” Analytics is about “anticipate and shape” models. Predictive analytics is about finding a needle (nugget) in a haystack of data with processing being done in real-time in the cloud.
There is no dearth of data. However, for BI or Analytics use cases to work, they must first be based on high-quality data and not merely on quantity. In other words, not all the data any given company possesses has worth, and not all of it is dependable enough to be of use. Hence the data supply chains (also called information logistics), both internally and externally, are becoming more critical in various enterprise strategies.
Another interesting angle is that with every technology cycle… Reporting, Visualization, Dashboards and Analytics platforms have evolved to fit/match. The market leaders of one generation were rarely the leaders in the next generation. We are at the cusp of another major Wave 4 upheaval as we move towards mobility and sensors as a dominant paradigm. The new wave includes (1) devices acting as sensors for intelligent data collection; (2) devices whose UI is on the web rather than the device; (3) sensors feeding data into multiple online services setting the stage for augmented reality, personal intelligence and decision management; and (4) crowdsourcing which includes the use of humans as sensors.
How Important is BI and Analytics to C-Level Executives?
“It took 50 years for organizations to fully leverage transactions systems, so it will time to realize significant value from knowledge, information and data.” — Peter Drucker
Who doesn’t want this…. The intelligent use of information to improve investment decisions, operational performance and customer outcomes. This is the reason why BI and Analytics is ranked consistently in the top 2 priorities for CIO and business leaders in every survey. The importance of BI and Analytics is growing every year in every company.
The future of BI is evolving in both worlds… enterprise AND consumer. The focus for CIOs is shifting from support decision making TO Disrupt with next gen apps; Disrupt with breakthrough technology; Disrupt with a revolutionary business model.
The old paradigm… a world in which analysts and executive study data and make decisions. The new paradigm… analysts study data, develop models and write algorithms that make automated decisions.
Most organizations and CIOs are not ready to for this radical shift. Real-time, location-driven, multi-channel, peer-to-peer interactions, conversations are no longer buzzwords but new corporate reality.
See this top 10 CIO Business and Technology Priorities (Source: Gartner). Not much changed in 2014. Most organization’s infrastructure, operations and scientific skills have not caught up to Big Data.
However, the pace of change and transformation is uneven and varies by industry and specific function (regulated vs. non-regulated). So context is important. Going from the industry to your company/organization…. specifically, what are you trying to accomplish?
- Spot trends and anomalies in business data
- Conduct deep trend analyses using statistical and financial performance management software
- Perform “what if” analysis and predictive modeling to predict potential threats and opportunities
- Facilitate accurate, timely financial and regulatory reporting for proactive planning and budgeting
- Allow executives greater visibility into operational, financial and market risk
If you said “yes” to any of the above then you are a candidate for BI and/or Analytics solutions. The challenge is institutionalizing these into repeatable capabilities.
What is Business Intelligence (BI)?
Measure what matters, while what matters is changing.
BI is the techniques used in spotting, digging-out, and analyzing ‘hard’ business data, such as sales revenue by products or departments or associated costs and incomes. Objectives of BI implementations include
- understanding of a firm’s internal and external performance against Key Performance Indicators (KPIs);
- understanding of the relationship between different data for better decision making;
- detection of opportunities for innovation, and
- cost reduction and optimal deployment of resources.
Most widely used BI tool is Microsoft Excel.
Business Intelligence:
- focus is on retrieval and delivery of data
- monitoring and identifying exceptions
- limited variability, ambiguity, uncertainty
- reporting, dashboards, scorecards, OLAP for bounded exploration and analysis
Business intelligence software allows companies to tap into their many databases and deliver easy‑to-comprehend insight to employees, management, and business partners. The focus is on answering “how am I doing”, “why”, and “what should I be doing?”
BI software — Query, reporting, analysis, scorecards and dashboards — is already being used by thousands of companies to find new revenue opportunities, reduce costs, reallocate resources, and improve operational efficiency.
What does BI at Apple look like? Apple’s Information Services and Technology department operates a Teradata enterprise data warehouse, along with Oracle databases. Apple uses “extract transform load”(ETL) and data integration tools from Informatica and other providers deliver access to multiple terabytes of data from SAP enterprise resource planning (ERP) software and other data sources. These provide reporting solutions for the company’s cross-functional business units, including marketing, sales, operations, support and finance.
What is Business Analytics?
Analytics is the extensive use of data, statistical and quantitative analysis, explanatory and predictive models, and fact-based management to drive decisions and actions.
Business Analytics
- Innovate through Decision Process Reengineering…. focus is on generation of new data, insight/foresight
- exploring data, finding insights
- expect uncertainty and probability and pattern rather than specific data
- computational and probabilistic techniques
Business analytics is about “anticipate and act” to drive Better Outcomes, Smarter Decisions, and Actionable Insights. Analytics has been defined as “the extensive use of data, statistical and quantitative analysis, explanatory and predictive models, and fact-based management to drive decisions and actions” (Davenport and Harris, Competing on Analytics, 2007). Analytics is an umbrella term that encapsulates data collection, statistics, data mining, predictive modeling, and decision sciences.
There are three types of data analysis:
- Predictive (forecasting),
- Descriptive (business intelligence and data mining) and
- Prescriptive (optimization and simulation)
See Predictive Analytics 101 for a quick overview. Data analytics software and advanced analytics techniques, include predictive analytics, text analytics and text mining, customer analytics and data mining.
Analytics is growing exponentially in competitive segments like consumer marketing. For example, NetFlix mines its video rental history database to recommend rentals to individual customers. American Express can suggest products to its cardholders based on analysis of their monthly expenditures.
A widely quoted example of predictive analytics insight is the Diapers and Beer sales co-relation. A grocery chain used Oracle BI to analyze local buying patterns. They discovered that when men bought diapers on Thursdays and Saturdays, they also tended to buy beer. Further analysis showed that these shoppers typically did their weekly grocery shopping on Saturdays. On Thursdays, however, they only bought a few items. The retailer concluded that they purchased the beer to have it available for the upcoming weekend. The grocery chain could use this newly discovered information in various ways to increase revenue. For example, they could move the beer display closer to the diaper display. And, they could make sure beer and diapers were sold at full price on Thursdays.
What is Big Data?
We’ve entered a new phase in the “industrialization of data.” We are addressing peta-byte scale problems.
The big data analytics opportunity can be summarized as:
- We have a wealth of data/content, Petabytes
- We have broad varieties of data
- We have varying velocities and arrival rates
- Traditional methods cannot keep up with the “3 V”s
Big Data is… a catchall term referring to technologies and initiatives that involve data that is too diverse, fast-changing or massive for conventional technologies, skills and infrastructure to address efficiently.There are leverage opportunities in a variety of industries around: Manufacturing, Supply Chain, Customer Facing and Consumer Facing processes.
The consumer generates incredible amount of data as they shop, browse, click, comment, game on the Web. Credit card transactions, UPC barcode reads, RFID scans and GPS location data all add even more data. Piles of data is being created by every second sensors from traffic, heating, ventilation and air control (HVAC) and industrial plant monitoring to automotive sensors .
For this streaming or event processing data, individual packets may be quite modest in size but start to become “big data” when aggregated and analyzed over many days, months and years.
What is Big Data Analytics?
The problem often isn’t finding data (search), it’s figuring out what to do with it and how to turn it into “relevant information”. This is the essence of data science and analytics. See this blog posting on my views of big data use cases.
A very good example of data science and analytics can be seen at Facebook. Facebook builds a custom Web page every time you visit. It pores over all the actions your friends have taken—their postings, photos, likes, the songs they listen to, the products they like—and determines in milliseconds which items you might wish to see, and in what order. Is this the future for every firm?
Consider how Lufthansa is innovating around revenue management – pricing and capacity management. It’s running a pilot project with SAP’s HANA. HANA’s in-memory technology allows Lufthansa to load more data and application functionality into memory—rather than from disk— dramatically improving application performance. Revenue optimization calculations are now done in real time, rather than in batch mode. The application aggregates more data than previous models (e.g., passenger data over two years). The system calculates and optimizes the revenue for origin/destination (OnD) itineraries, and bases pricing on passenger profiles. It also estimates the likelihood of cancellation and no-shows on flights, and thus how much overbooking to allow.
Big data analytics is a technology-enabled strategy for gaining richer, deeper, and more accurate insights into customers, partners, and business operations. This collection of tools, techniques, and technologies provide insights from complex, large data sets. By processing a steady stream of real-time or static data, organizations can make time-sensitive decisions faster, monitor emerging trends, course-correct rapidly, and jump on new business opportunities.
Two significant technological advances have converged to unlock the value of data for organizations. Fueled by increasing data volumes and throughput, they have co-evolved to empower developers and data scientists to navigate vast data collections and discover new business models or customer segments.
The first component is the introduction of software frameworks, such as the Apache Hadoop framework, which allow data to be easily stored retrieved and queried at scale by distributing it across a number of different computers and disk. Such tasks would have taken many skilled computer scientists months to concoct only a few years ago; today, getting up and running with a distributed data platform is quick and easy with the Hadoop framework, since the software handles most of the complexity.
The second advance is cloud computing. The success of analytics software and the growth of the Hadoop ecosystem would not been for the dramatic increase in availability of infrastructure to store and query that data, in the form of cloud computing. The ability to do fast provisioning of scalable, secure infrastructure capable of managing modern data, available to anyone at a fraction of the traditional cost is critical for test-and-learn innovation.
Building Big Data solutions requires making a lot of choices. Our friends at IBM have a great way of illustrating this.
Big Data – Hype/Fatigue vs. Reality
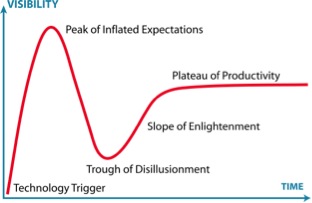
With so many different interpretations, pinning down true value is difficult. As a result, there a backlash brewing and fatigue is setting in. Business and IT executives have begun to express skepticism indicative of early disillusionment in the hype cycle….the message…. Don’t darken our doors selling Big Data.
We have seen this cycle before with multiple “savior” technologies. We are yet to reach the trough of disillusionment yet. See this blog posting on my views of big data startup failures and consolidation.
BI or Analytics – Where should you focus?
The line between BI and Analytics is rapidly getting blurred. However, some authors view analytics as a subset of business intelligence (BI): “a set of technologies and processes that use data to understand and analyze business performance ” and “includes both data access and reporting, and analytics” (Davenport and Harris, Competing on Analytics, 2007).
WalMart, for instance, does BI and Analytics well. Retail Link is Walmart’s online warehouse for sharing up-to-date point-of-sale information with suppliers. WalMart captures point-of-sale transactions from over 3,900 stores and continuously transmits this data to its massive 10+ petabyte Teradata data warehouse. WalMart allows suppliers, to access data on their products and perform data analyses. These suppliers use this data to identify customer buying patterns at the store display level. They use this information to manage local store inventory and identify new merchandising opportunities. Back in 2007, Retail Link tracked some 800 million transactions per day, with detail down to the store and item level.
So what fundamental tools and techniques are embedded in Retail Link…
- Forecasting – leveraging historical data to drive better insight into decision making for the future
- Optimization – analyze massive amounts of data in order to accurately identify areas likely to produce the most profitable results
- Data mining – mine transaction databases for data on spending patterns such as indicate a stolen credit card
- Text analytics – finding value in unstructured data like social media that could uncover insights about consumer sentiment
Definitions of Different Analytics and BI Categories
- Corporate/enterprise performance management software and performance management concepts, such as the balanced scorecard, enable organizations to measure business results and track their progress against business goals in order to improve financial performance.
- Business intelligence (BI) is a necessary business competency for improving decisions and performance. the most widely used BI tool is the spreadsheets. Traditionally, BI has been used for performance reporting from historical data, and as a planning and forecasting tool for a relatively small number of people in an organization. Modeling future scenarios permits examination of new business models, new market opportunities and new products, and creates a culture of opportunity.
- Data visualization tools, include mashups, executive dashboards, performance scorecards and other data visualization technology, is becoming a major category. In general, one good data visualization can put many ongoing verbal arguments to rest.
- Data analytics software and advanced analytics techniques, including predictive analytics, text analytics and text mining, customer analytics and business intelligence – customer, supply chain – data mining, can help organizations make sense of — and gain a competitive advantage from — all the data that they have in their systems.
- BI platforms provide a range of capabilities for building analytical applications. Examples are Oracle OBIEE, SAP Business Objects 4.0. There are many choices and combinations of BI platforms, capabilities and use cases as well as many emerging BI technologies such as in memory analytics, interactive visualization and BI integrated search. The idea of standardizing on one supplier for all of one’s BI capabilities is difficult to do. Increasingly, standardization and more about managing a portfolio of tools used for a set of capabilities and use cases.
- Data integration tools and architectures in support of BI continue to evolve. Extract-Transfer-Load (ETL) tools make up a big segment of this category in addition to data mapping tools. Organizations must now support a range of delivery styles, latencies, and formats.
- Data Cleansing. The first step of any data analysis project is organizing and cleaning the data… basically “data conditioning,” or getting data into a state where it’s usable.
- Data Virtualization deals with an abstraction layer of information from many disparate data sources — so it can integrate with data tied to applications, databases, files, virtualization, clouds etc. A key component in any large data virtualization implementation is the connectivity and data transfer layer that ensures consistent performance while accessing all of the disparate data, especially if the data is located across many servers, clouds and virtualized platforms.
BI and Analytics Stack
Information Governance and Analytics Tools
Cloud. On-Premise. Hybrid IT. Companies have to restructure and reorganize to manage data. Most organization’s infrastructure, operations and skills haven’t evolved to catchup with analytics and Big Data.
Traditionally most firms have focused their efforts on solutions within business units leading to data silos across the enterprise. With data increasingly critical to business strategy, the problems of poor quality data, fragmentation, and lack of lineage are also taking center stage.
Establishing an enterprise information framework to support the business is pretty much every CIO’s top objective. Information governance is the organizational linchpin needed to build out foundational competencies, processes, policies, measurements, and roles and responsibilities to finally make valuable enterprise data more useful.
Better coordination to manage, govern and secure the data, tools and skills is a must-have. We anticipate that spending on tools and management techniques will increase substantially from current levels in the next 5 years.
Notes and References:
- IBM Patent — System and method for delivering business intelligence data in a client/server architecture (US 7783724 B2)
- Systems and methods for providing custom or calculated data members in queries of a business intelligence server – US8458206, Jun 2013; Oracle Corporation.
- Mobile BI Patent for Unisys Corp. — System and wireless device for providing real-time alerts in response to changes in business operational data
- http://www.slideshare.net/timoreilly/the-future-of-business-intelligence
- Big Data – when volume, velocity and variety of data exceeds an organization’s storage or compute capacity for accurate and timely decision making. With the addition of “Big Data” to the Oxford English Dictionary in June 2013, the term has officially gone mainstream.
- With Advanced Analytics Magic Quadrant, Gartner reveals a new guard of companies challenging IBM and SAS. Alteryx, Revolution Analytics, RapidMiner and Knime are the ones to watch in 2014.
Related articles
- Predictive Analytics 101 (quick overview)
- Executing Analytics 101 (quick overview)
- Market Size of BI and Analytics
- IBM CIO Study: BI and Analytics are #1 Priority for 2012 (practicalanalytics.wordpress.com)
- Big Data and Hadoop – Investment Theme
- Big Data According to Goldman Sachs
- The Heritage Heath Prize: Find out more about the highly publicized contest that aims to identify who will be admitted to a hospital within the next year, using historical claims data.
- Predictive Analytics: Read the Wikipedia page on predictive analytics for an overview of common applications and techniques used to make predictions about the future.
- Data Mining: Find more about this topic in Wikipedia.
- Hadoop gets real — Computerworld details how a number of companies are benefiting from Hadoop’s ever-increasing capability and functionality, and improved ease of use. The article focuses on an interview with Inovalon’s CTO, who discusses how the company is leveraging Hadoop in detail.
- OkCupid’s Co-Founder on the Myth of the Data Scientist ‘Unicorn’ — The Wall Street Journal interviews OkCupid founder Christian Rudder, who defended Facebook’s user sentiment experiments on the company’s blog in July. Rudder aims to dispel a number of persistent myths and misconceptions about the discipline, explaining OkCupid’s rationale behind its own user experience experiments, and stating that while data science requires a number of specialized skills, practitioners need not be ‘special geniuses’ to be effective data scientists.
cr:https://practicalanalytics.wordpress.com/bianalytics-basics/
ไม่มีความคิดเห็น:
แสดงความคิดเห็น